AI in radiology: here to stay
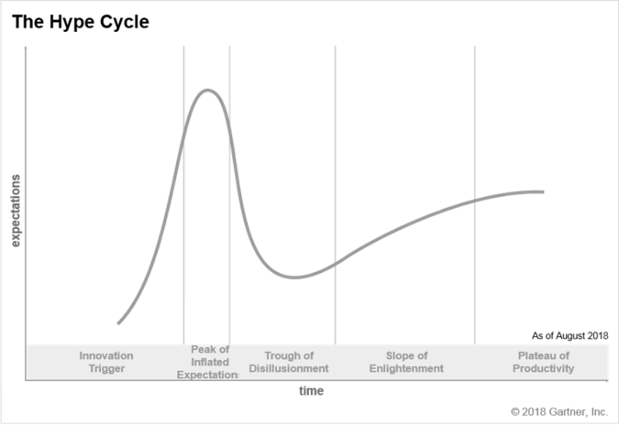
It also brings to mind the multidisciplinary approach required to develop new AI methods, a concept that surfaced at ECR 2022 as well as other recent conferences we attended, including the Annual Meeting of the American Society of Neuroradiology (ASNR). Expertise across radiology, neurology, physics, statistics, and machine learning domains is needed and as early as possible in the development of an AI model. This multifaceted domain knowledge helps answer questions such as:
- What are the pain points being experienced by the end users?
- What would they like to be able to accomplish?
- Which features would make their job more efficient or provide insights they weren’t able to achieve previously?
- What is possible with existing technology?
- How do we ensure it is accurate and valid within this context?
- Which datasets do we need to train and develop new models?
Getting the right people in the room early can help make sure that the technology will be valuable, accepted, and trustworthy — minimizing the need for costly, time-consuming changes after the technology has been deployed.
Moving past the “black box”: gaining an understanding of AI
Trust in the output: new opportunities for value-added services
It is this type of transparency in our platform and its reporting that has contributed to end user confidence in its predictions. We heard firsthand at the conference that some attendees previously considered our products helpful only for patients with dementia; however, after viewing our clinically focused reports for MS, epilepsy, and traumatic brain injury (and the methodology behind them), they were interested in expanding the breadth of patients they could support using our platform. This feedback extends to our Dementia Differential Analysis report, which we presented at the Artificial Intelligence Theatre. The value of the report continues to be viewed positively, especially since the contribution of each imaging biomarker to the predicted diagnosis is openly available for review. Watch the video below to learn more about the imaging biomarkers and how they’re used to determine the most probable dementia diagnosis.