More than 55 million people, worldwide have been diagnosed with dementia, with approximately 10 million new cases annually. An early and precise dementia diagnosis is essential to providing the best possible care and advance therapeutic research. As such, it is important to distinguish between the many types of dementia, including Alzheimer’s disease (AD; the most common type), Lewy body dementia, frontotemporal dementia (FTD), vascular dementia, and others.
Although detection of and differentiation among the various types remain challenging for clinicians, technological advances such as artificial intelligence (AI) and machine learning (ML) can help. We discuss how this technology can be applied both in our webinar, Making data meaningful: data-driven diagnostics in neurodegenerative disorders, and in this blog post.
Challenges the clinician faces
With advances in disease understanding, imaging capabilities, and tests for genetic and other biomarkers, a more comprehensive view of a patient’s condition has become possible. The ability to pinpoint underlying abnormalities is transforming dementia care and management, particularly in light of emerging treatment options and disease-modifying therapies. However, the amount of data available for a given patient is also overwhelming clinicians’ abilities to accurately collate and understand those data in a timely manner.
Types of data that can be available for patients:
- Magnetic resonance imaging (MRI)
- Positron emission tomography (PET) imaging
- Cerebrospinal fluid (CSF) biomarkers
- Neuropsychological test results
- Other demographic and clinical characteristics
- Genetic information
At the same time, for some patients, information from additional tests could be beneficial for differential diagnosis and long-term monitoring. However, given the cost and burden of these tests, such as additional imaging or CSF testing, clinicians want to be confident that the results will truly be beneficial but are challenged by knowing which tests will be useful, at what time, and for which patients.
Compounding this is the difficult task of extracting all the relevant information from available imaging. Brain imaging plays a key role in distinguishing subtle differences between healthy and pathological volume loss and between dementia subtypes, particularly when there is some overlap between clinical symptoms (e.g., between FTD and AD). Yet, it is these subtle, but important, differences that are difficult to assess on images by visual inspection alone and require training and expertise.
Automated MRI quantification
As we understand more about how disease-related mechanisms manifest as volumetric differences in key brain structures (e.g., hippocampus, ventricles) and lesions, image analyses to detect and quantify these differences have become increasingly important. Imaging biomarkers derived from brain volumetry in dementia, such as hippocampal volume, can support early detection, differential diagnosis, and tracking of disease progression.
Some distinguishing patterns of neurodegeneration include mediotemporal (including the hippocampus) and parietal atrophy in typical AD, atrophy focused in the frontal and temporal lobes in FTD, and white matter hyperintensities (WMHs) in vascular dementia. These patterns of neurodegeneration can be assessed visually, but this is difficult, especially for early cases.
In contrast, automated imaging quantification using AI technology can improve the objectivity and consistency of image interpretation, even for less-experienced readers. These methods are based on brain segmentation to provide region-specific volumetric measurements that can be compared with normative populations as well as for the same patient over time. The comparison against a cognitively normal data set helps distinguish between pathogenic patterns and age-related changes (e.g., more abundant WMHs and reduced hippocampal volume).
Earlier detection of abnormalities and greater differentiation have been demonstrated with automated quantification, when compared with manual visual reads. Further, technology-enabled assessments can compute multiple scales in a short period of time, arming the clinician with the information needed for more confident diagnoses.
Combining patient data in a meaningful way
However, providing clinicians with additional and more objective data doesn’t solve the issue of information overload. To address this challenge, a comprehensive technology solution is needed to:
- Systematically combine and visualize all the relevant patient data
- Provide an overview of the patient’s condition
- Be able to handle conflicting and missing data
- Accurately compare different types of dementia
Based on our own experience and ongoing collaborations with clinicians, we developed cDSI™, part of our cNeuro® suite of products. By combining key patient data from multiple sources including automated MRI quantification from our cMRI™ application, cDSI provides clinical decision support for early, differential diagnosis; prediction of disease progression; and decisions about what testing is needed in patients with neurodegenerative diseases.
The proprietary disease state index (DSI) classifier in cDSI compares patient data to a high number of previously diagnosed cases. It determines how similar the patient’s profile is to AD, FTD, vascular dementia, and patients found out to have normal cognition (subjective memory complaints).
For this patient, the data fit best with vascular dementia, which is shown by the high DSI of 0.75 and the color red. The second-best fit is AD. However, the probability of correct class (PCC) value, or the probability that the diagnosis with the highest DSI value is correct, is 65%, which is not very high and does not provide confidence in making a diagnosis.
It is helpful that the fingerprint visualization provides insight into how this conclusion was reached, making the ML more transparent. In addition to the red and blue colors, boxes show the contribution of the specific determinant to the prognosis; the larger the box, the greater the contribution. In addition, the more vivid the color, the more likely the diagnosis. So, we can understand the data supporting the DSI and PCC.
Yet, the data do not support a definitive diagnosis. In this case, more data might be useful. CSF testing would help determine the levels of biomarkers such as amyloid and tau. Although appropriate use criteria for the optimal use of CSF biomarkers state that CSF testing can be performed in all patients with suspected AD, this is not really feasible in clinical practice, especially given its high cost and invasiveness. Therefore, clinicians would benefit from some guidance to know whether CSF testing would help with a more accurate diagnosis.
Stepwise approach to making decisions about testing
To help inform testing decisions such as whether the cost:benefit ratio of CSF testing is appropriate for a specific patient, a stepwise, data-driven approach to testing is used by our cDSI application:
- Measure the DSI and PCC.
- When the diagnosis is not confident enough, add simulated CSF values, both for positive AD and negative control CSF results.
- When the simulated CSF values result in an increased PCC, perform a lumbar puncture for CSF.
This approach was tested in more than 500 patients in in a recent study. The outcomes were compared with two other approaches:
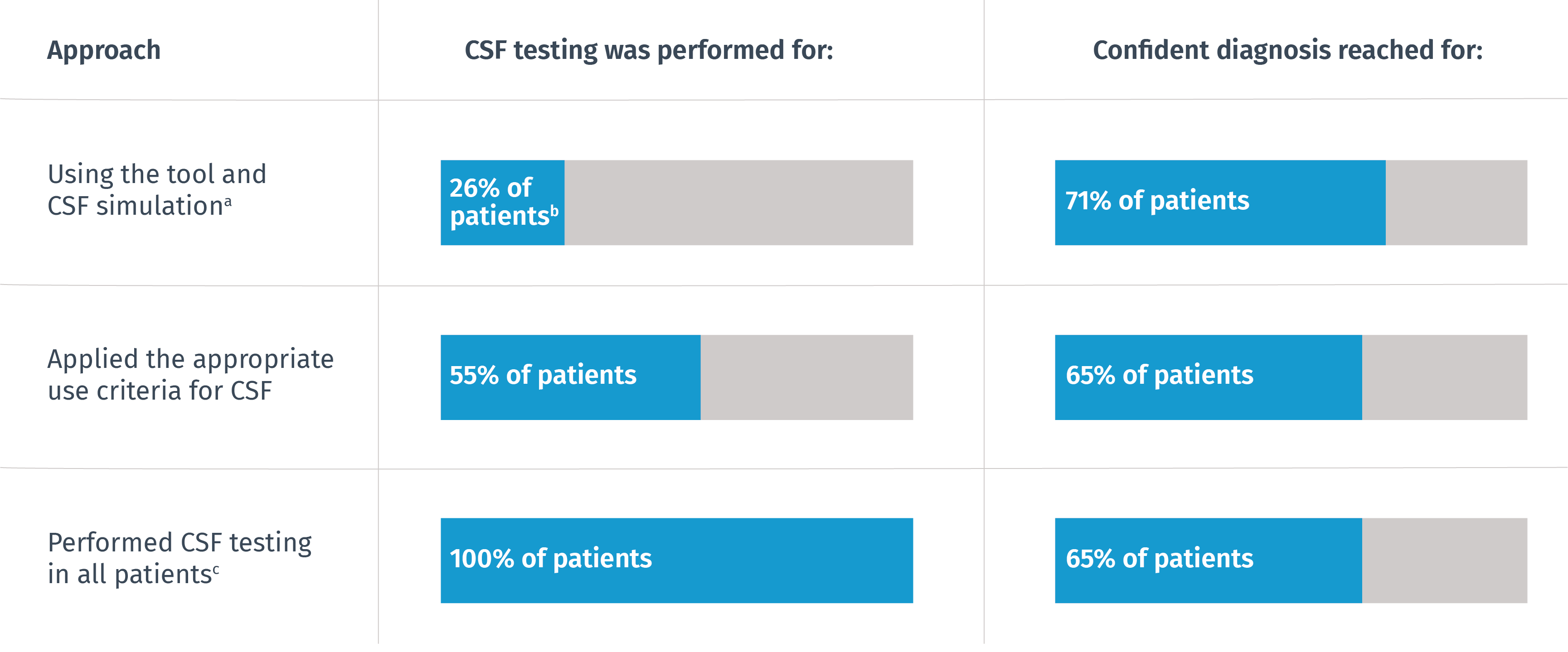
aWith tool alone, 58% of patients had a confident diagnosis.
bFor these patients, simulated CSF values increased the confidence, so testing was performed.
cDiagnosis was based on neuropsychology, MRI, and CSF.
bFor these patients, simulated CSF values increased the confidence, so testing was performed.
cDiagnosis was based on neuropsychology, MRI, and CSF.
The technology-supported approach yielded the largest proportion of diagnoses with sufficient confidence, with CSF testing suggested for only
26%
of the cases.
Artificial intelligence in clinical practice
To support the clinician, an AI-driven tool should comprehensively combine and visualize all the data, provide an overview of the patient’s condition, manage missing data, and fully automate MRI analysis. Further, an AI tool can help the clinician differentiate between several pathologies and simulate test results to guide clinicians in decisions about which tests to conduct.
With access to AI-driven tools, clinicians can derive meaning from greater amounts of data than ever before, allowing them to confidently choose the most appropriate diagnosis for each patient. In this way, clinicians have the ability to improve and personalize patient care, treating the patient’s memory disorder with precision as unique as the patient themselves.
Watch our webinar, Making data meaningful: data-driven diagnostics in neurodegenerative disorders, to learn more about the application of AI for differential diagnosis in dementia, and contact us to schedule a demo of our cDSI application.