What is it about AD that makes drug development challenging?
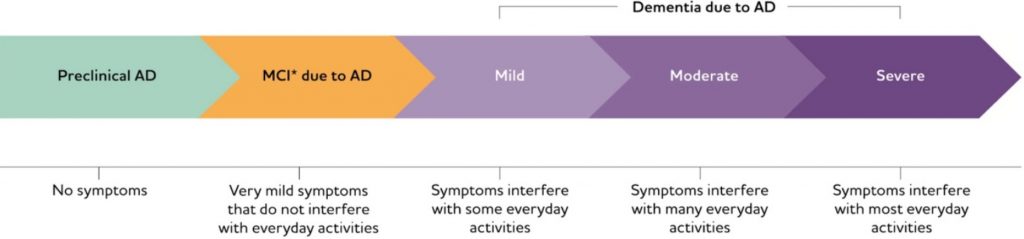
Why is the right patient selection for Aduhelm and drugs in clinical practice and clinical trials important?
One reason for trial failure is inappropriate patient selection. In many studies, the drug was administered too late in the disease course, or the sample was too heterogeneous. In other studies, the study duration was too short to prove a drug effect. In some cases, the need to reduce the cost of a lengthy trial could have contributed to shortened timelines.
based on 540 patients in three memory clinics in the Combinostics database
How can we ensure appropriate patient selection for Aduhelm and other DMDs in development?
The specific diagnosis (AD vs other forms of dementia) and likely progression can be determined by providing the machine learning model with all of that patient’s available data, against which it can compare with the database of previous patients’ data.
For DMDs:
If amyloid results are available, they can be included in the predictive analytics to determine whether the patient is on a slow or fast track for cognitive decline.
For clinical trials:
This type of system could help identify patients who have the required characteristics (i.e., amyloid plaque) and are likely to respond to the drug, reducing the “noise” associated with disease heterogeneity.
It can provide evidence for the suitability of that drug for the specific patient.
When multiple DMDs against AD are available on the market, it can be used to decide which drug is likely to work best for each patient.
So then, our answer to the question posed in the title is, quite simply, yes. The use of AI for patient selection is not a pie-in-the-sky idea — the capabilities already exist in our cDSI product, which is part of the cNeuro platform. We’re certain that AI can — and will — help pharma companies select and monitor trial participants during drug development, as well as help clinicians decide whether a patient is eligible for a certain type of treatment.
References
- Matthews, K. A., Xu, W., Gaglioti, A. H., Holt, J. B., Croft, J. B., Mack, D., & McGuire, L. C. (2018). Racial and ethnic estimates of Alzheimer’s disease and related dementias in the United States (2015–2060) in adults aged≥ 65 years. Alzheimer’s & Dementia 15(1):17-24. https://doi.org/10.1016/j.jalz.2018.06.3063
- Wong, W. (2020). Economic Burden of Alzheimer Disease and Managed Care Considerations. Am J Manag Care 26:S177-S183. https://doi.org/10.37765/ajmc.2020.88482
- Gauthier, S., Rosa-Neto, P., Morais, J.A., & Webster, C. 2021. World Alzheimer Report 2021: Journey through the diagnosis of dementia. London, England: Alzheimer’s Disease International.
- Atri, A. (2019). Current and Future Treatments in Alzheimer’s Disease. Semin Neurol 39(2):227-240. https://doi.org/10.1055/s-0039-1678581
- Food & Drug Administration. (June 7, 2021). FDA Grants Accelerated Approval for Alzheimer’s Drug. Available at: https://www.fda.gov/news-events/press-announcements/fda-grants-accelerated-approval-alzheimers-drug